Formatting biological big data for modern machine learning in drug discovery
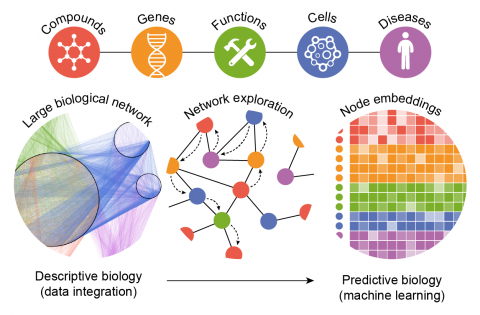
Duran‐Frigola M, Fernández‐Torras A, Bertoni M, Aloy P, Biological data is accumulating at an unprecedented rate, escalating the role of
WIREs Computational Molecular Science,
2018
data-driven methods in computational drug discovery. This scenario is favored by
recent advances in machine learning algorithms, which are optimized for huge
datasets and consistently beat the predictive performance of previous art, rapidly
approaching human expert reasoning. The urge to couple biological data to cutting-
edge machine learning has spurred developments in data integration and knowl-
edge representation, especially in the form of heterogeneous, multiplex and
semantically-rich biological networks. Today, thanks to the propitious rise in
knowledge embedding techniques, these large and complex biological networks
can be converted to a vector format that suits the majority of machine learning
implementations. Here, we explain why this can be particularly transformative for
drug discovery where, for decades, customary chemoinformatics methods have
employed vector descriptors of compound structures as the standard input of their
prediction tasks. A common vector format to represent biology and chemistry may
push biological information into most of the existing steps of the drug discovery
pipeline, boosting the accuracy of predictions and uncovering connections between
small molecules and other biological entities such as targets or diseases.
Direct link: 10.1002/wcms.1408