Background
The SARS-CoV-2 outbreak has prompted an unprecedented effort by the scientific community to find an effective vaccine or drugs to mitigate the viral infectiveness and symptoms, which is reflected in the over 4,000 publications that appeared in the last weeks. The volume of information is inaccessible by any single research group, and this may limit progress towards the rapid discovery of a COVID-19 therapy. Through a review of the most relevant scientific literature, and considering different levels of experimental evidence, we have identified over 150 compounds that are potentially active against COVID-19.
We now exploit this literature curation effort to identify other compounds with the potential to be effective against COVID-19. To this aim, we use the (CC), a resource that provides processed, harmonized and integrated bioactivity data for about 1M small molecules. In the current resource, we provide the results of a systematic similarity search across the large chemical space encompassed by the CC, thereby substantially expanding the portfolio of potential COVID-19 drug candidates.
Strategy
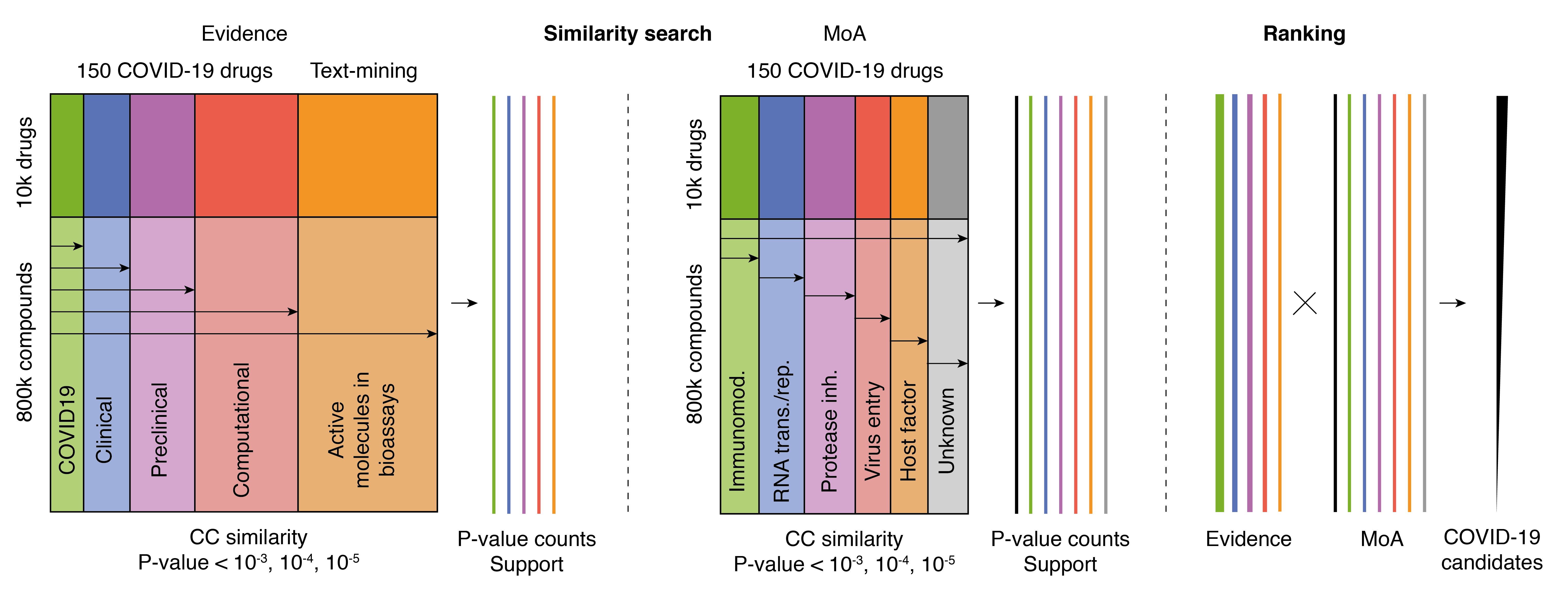
By mining the recent literature, we identified about 150 drug-like compounds that have been suggested by the scientific community to be potentially active against COVID-19. The scientific evidence supporting these compounds is variable: some of them come from computational predictions, some have proven their value in pre-clinical tests, others are approved drugs with a therapeutic annotation unrelated to infectious diseases and, finally, some are drugs currently used to fight related pathogens. As a community effort, we encourage researchers to include the compounds and/or anti-COVID-19 evidence that they are currently collecting. Additionally, we also include compounds that are positive in certain bioassays, identified as important through the automatic mining of the COVID-19 literature, and for which we find further bioactivity support in the CC. The whole pipeline described below will be automatically run every day, so that the resource always provides the most updated results.
Starting from the updated list of compounds, we run bioactivity and chemical similarity searches against the almost 1M compounds characterized in the CC. The results are weighted to favour molecules with similar properties to those with higher levels of experimental evidence and to ensure that the diverse mechanisms of action are represented. Finally, we collect and display the top 10,000 molecules, which can be sorted according to different criteria, including whether they are approved/experimental drugs, the cumulative level of support, or their similarity to literature drugs.
Query examples
The pre-computed similarity matrix can be queried to extract candidates that fulfill properties of interest. This can be achieved by selecting amongst the levels of evidence for the COVID-19 literature compounds as well as their mechanisms of action.
Below we show three exemplary queries highlighting 3 drug molecule candidates and 3 bioactive compounds from the larger chemical space of the CC.
Candidates similar to COVID-19 drugs with, at least, preclinical evidence.
inchikey | name | is_drug | evidence | moa | support | lpv_5 | lpv_4 | lpv_3 | top1_inchikey | top2_inchikey | top3_inchikey | top1_name | top2_name | top3_name | smiles |
---|
Candidates with similarities to host factor and virus entry mechanisms of action, including computational evidence.
inchikey | name | is_drug | evidence | moa | support | lpv_5 | lpv_4 | lpv_3 | top1_inchikey | top2_inchikey | top3_inchikey | top1_name | top2_name | top3_name | smiles |
---|
Candidates with similarities to immunomodulators, protease inhibitors and RNA transcription/replication modulators.
inchikey | name | is_drug | evidence | moa | support | lpv_5 | lpv_4 | lpv_3 | top1_inchikey | top2_inchikey | top3_inchikey | top1_name | top2_name | top3_name | smiles |
---|
Statistics
The figure below quantifies the number of COVID-19 literature compounds available at each level of evidence (upper left) and MoA (bottom centre), together with their intersections (bottom left). The upper centre panel plots the distance of CC signatures between COVID-19 compounds (k nearest-neighbours; k=1-5). This plot shows that annotated COVID-19 compounds are considerably diverse.
The heatmap on the right displays a chunk of the similarity matrix, corresponding to the top 2,500 candidates ranked by support (no evidence or MoA filters). Coloured dots indicate significant similarities, and colours correspond to the levels of evidence.
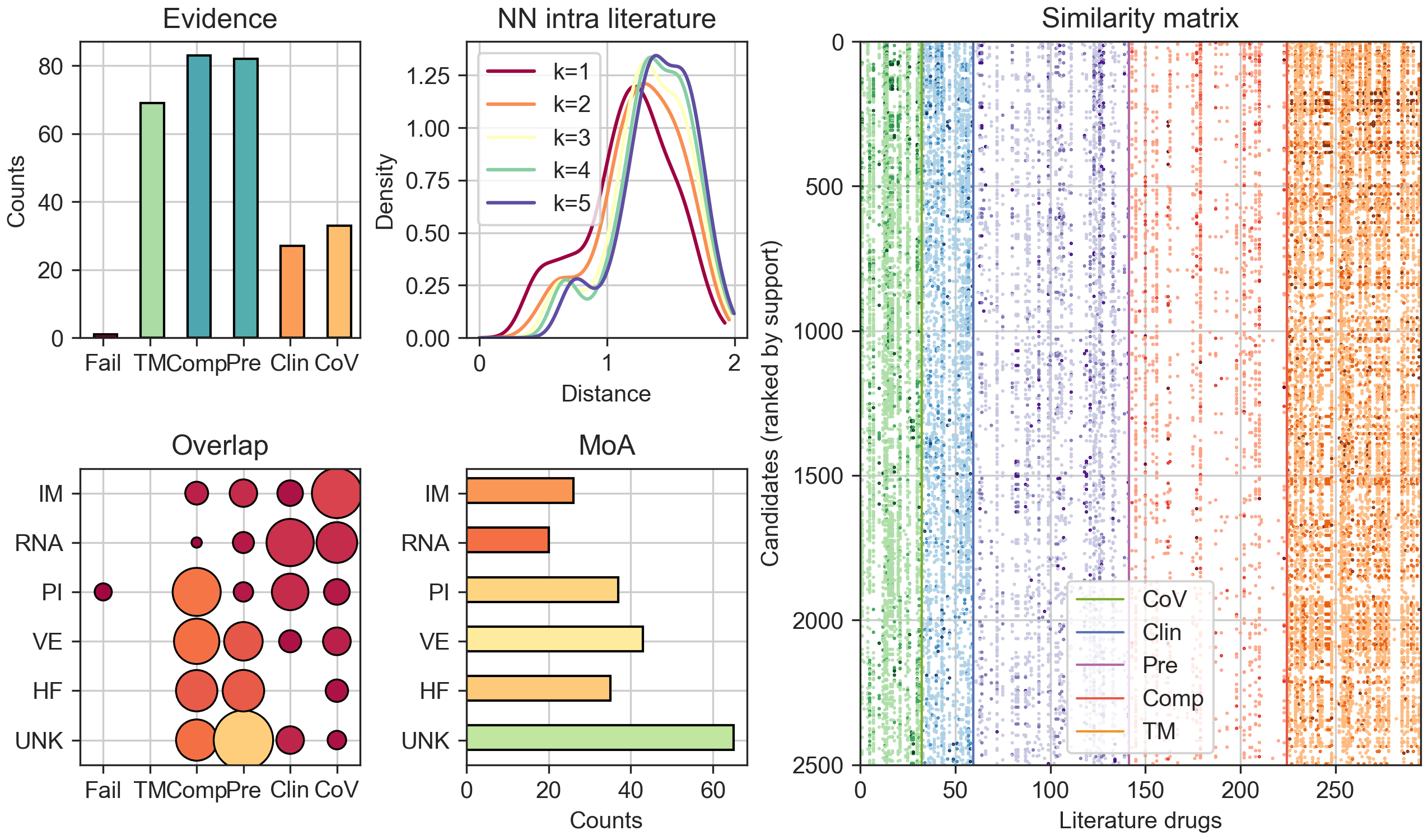
CC signatures can be projected on a 2D plane to obtain a global view of the chemical space explored by our resource. Upper panels project the COVID-19 literature drugs on the global space encompassed by the CC. As can be seen, COVID-19 compounds, while significantly diverse, cluster in certain regions of the chemical space. Bottom panels display the top 10k candidates (ranked by support) together with the current COVID-19 drugs. When these are labelled by MoA (bottom left), MoA-specific regions are revealed (e.g. host factor; HF), and candidates are retrieved in those regions. Overall, most of the COVID-19 drugs are ‘surrounded’ by new candidate molecules.
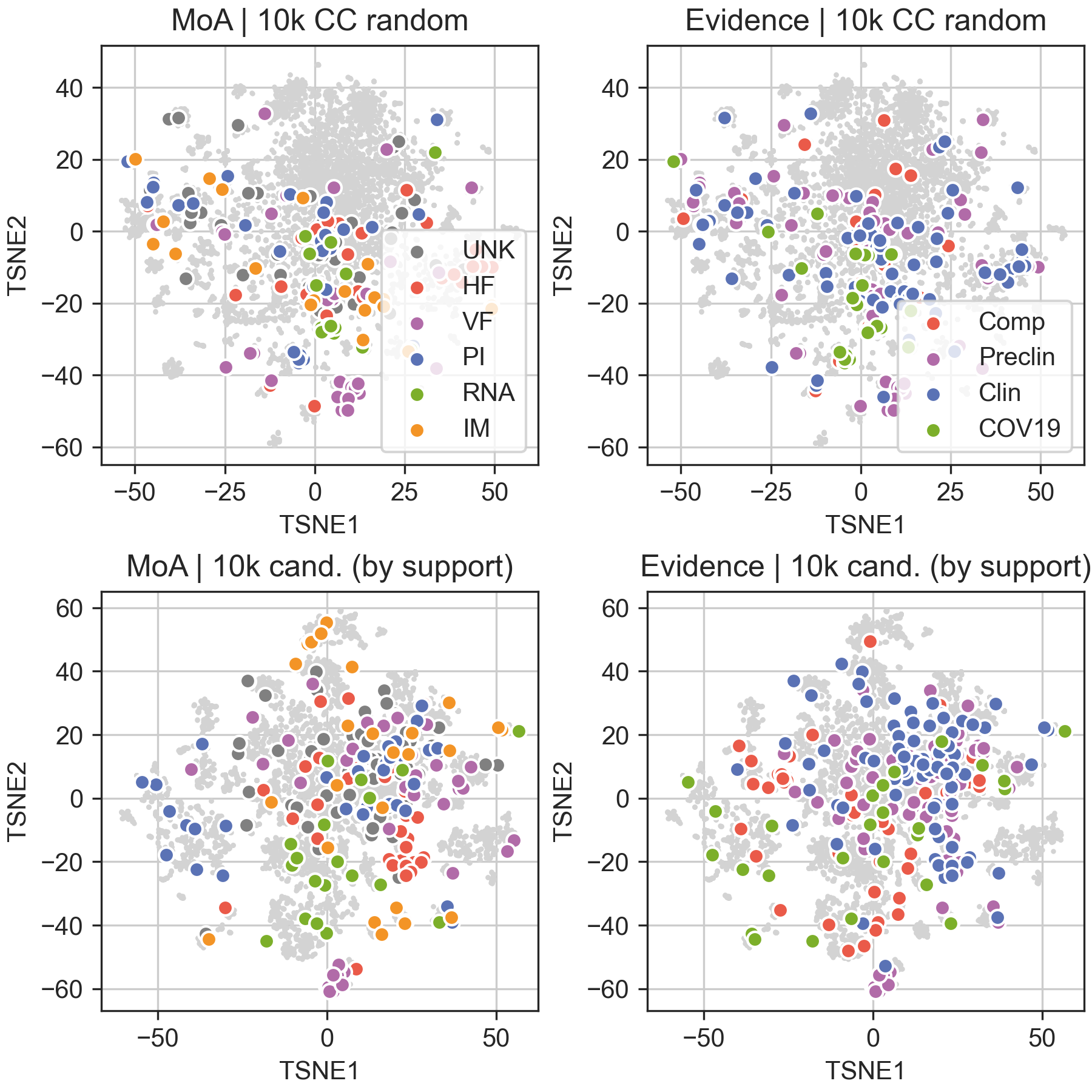
Reassuringly, when we analyze the therapeutic categories of the top-ranked candidates, as expected, we retrieve a significant number of antiinfectives and antiparasitic drugs. Of note, other therapeutic categories such as those related to hormonal treatments are enriched at after the highest-ranking compounds (right-most plot). Please note that, for this enrichment analysis, only drugs could be considered since ATC annotation are not available for most of the candidates.

To further validate our candidates, we checked, in a leave-one-out cross-validation, whether compounds at different levels of evidence (rows) could be retrieved by our similarity search using the COVID-19 literature drugs (columns). Indeed, the figure below shows that known COVID-19 drugs were significantly up-ranked (sum of supports) when using and evaluating all levels of evidence.
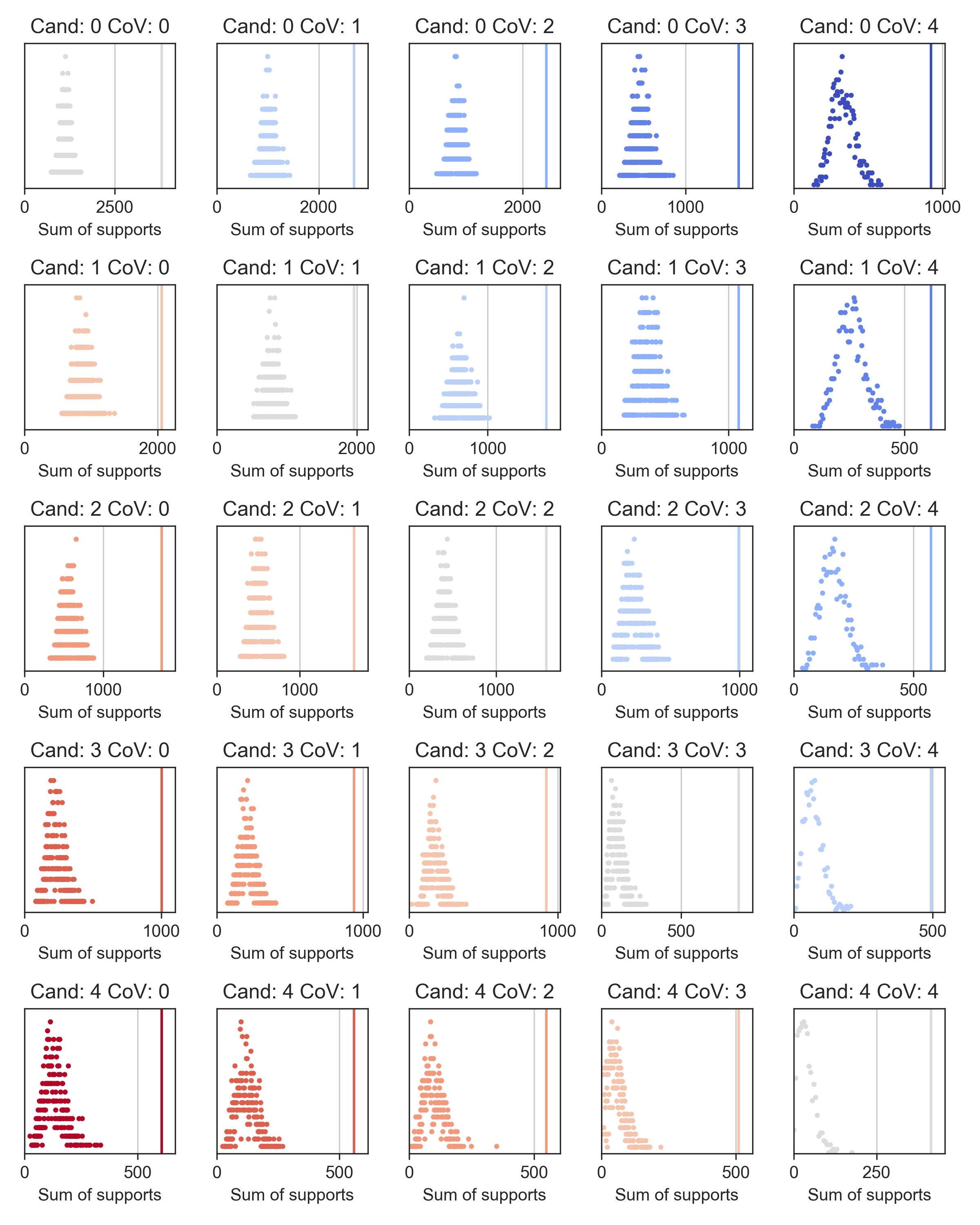
The statistical analysis presented herein suggests that our list of compounds significantly explores regions of the chemical space that could be relevant to COVID-19 treatment. We hope that our shortlisting of drugs and bioactive molecules will help expedite the discovery of a COVID-19 therapy.
Contributions
This resource has been developed by the Structural Bioinformatics and Network Biology Group at the Institute for Research in Biomedicine in collaboration with the Amazon Search Science and AI group on the NLP tasks.
IRB Barcelona Team: Miquel Duran-Frigola, Martino Bertoni, Eduardo Pauls, Víctor Alcalde, Oriol Guitart, Adrià Fernández-Torras, Lídia Mateo, Isabelle Brun-Heath, Núria Villegas, Carles Pons, Pau Badia-i-Mompel, Patrick Aloy.
Amazon Search Team: Roi Blanco, Víctor Martínez, Hugo Zaragoza.
We deeply thank all the medical staff for working to save our lives.